Dr
Alessandra
Mileo
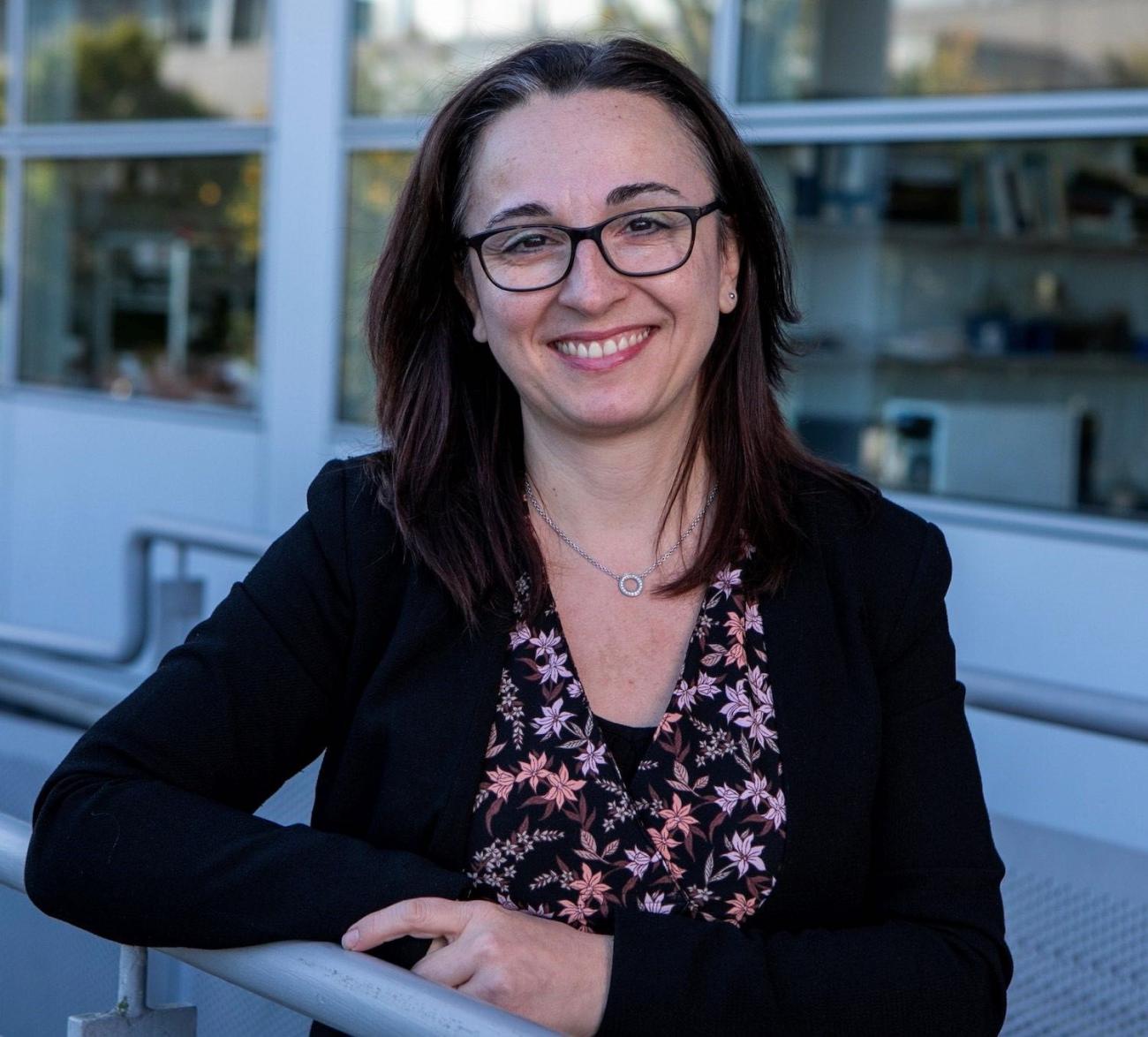
Academic biography
Prof. Alessandra Mileo is currently Associate Professor and Principal Investigator at the Insight SFI Research Centre for Data Analytics, Dublin City University (DCU). Before Joining DCU, she was a Senior Research Fellow, Adjunct Lecturer and Unit Leader at the INSIGHT Centre for Data Analytics, National University of Ireland Galway. She holds an MSC and a PhD in Computer Science from the University of Milan, Italy.
Before Joining the Digital Enterprise Research Institute (DERI, NUIG) in 2010, she was a Post-doctoral Researcher at the Nomadis Lab, Dept. of Informatics, Systems and Communication of the University of Milano-bicocca, conducting interdisciplinary research activities involving Ambient Intelligence and Knowledge-driven Sensor Fusion, which converged in the succesful EU project proposal EasyReach, AAL, 2009-2-17.
She continued her active contribution to successful EU proposals within DERI and later INSIGHT, acquiring a competitive EU project in the Smart Cities realm (CityPulse: Real-Time IoT Stream Processing and Large-scale Data Analytics for Smart City Applications) and she has been Principal Investigator for the primary industry collaboration within the Research Centre portfolio (Enabling the Internet of Everything: a Linked Data infrastructure for networking, managing and analyzing streaming information and follow up industry-funded projects).
She has been working for a few
Research interests
Previous research focused on Stream Reasoning. Current research focuses on Neurosymbolic AI, Explainability and Representation Learning.
In the Stream Reasoning area, I have been mainly working on scalable and interoperable ways of transforming web data streams into actionable knowledge. Research areas around this velocity aspect of Big Data involve distributed and parallel inference as well as the design of hybrid methods for qualitative and quantitative reasoning.Ongoing collaborations are currently in place on applying research outcomes in real world applications that have a very high economical and social potential impact. These include health monitoring, smart cities, sustainable IT and smart enterprise.In the areas of Neurosymbolic AI, Explainability and Representation Learning, I am interested in investigating new comprehensive approaches to data analysis that combine knowledge-driven and data-driven representations. Beyond the ability to crunch and learn from massive data, the new generation of intelligent machines will need to be able to explain their outcomes, debug errors and reduce data bias. Knowledge Representation, Rule Learning and Neural Networks can play a key role in designing machines that exhibit both cognitive and neural reasoning, but many open questions remain on how to most effectively combine these two capabilities. Along with these challenges, such new approaches will bridge the gap between two faces of AI (connectionist and symbolic) that are historically diverging.